Data Cleansing
Data Cleansing Services That Optimise Your Business Data & Maximise ROI
Data quality is a vital element to any business foundation, customer management and achieving the best possible outcomes. A typical database will go stale at 2% per month and up to 24% per year due to people moving, changing contact details, divorcing, dying and MORE. Melissa is proud to offer a comprehensive way to clean and maintain your customer data – a core component to any data management strategy.
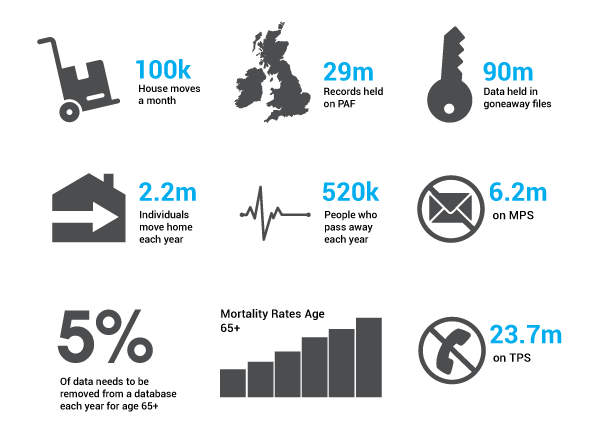
Address Verification
Verify, standardise, transliterate and correct addresses for 240+ countries and territories to ensure a clean and up to date address database.
Data Match, Merge & Deduplication
Uncover, merge, & purge duplicate records for a single customer view.
Preference / Opt Out Suppressions
Identify individuals with MPS and TPS preferences to ensure you maintain the preferred contact methods for your database.
Email Verification
Check and verify emails to improve deliverability, maintain spam compliance, reduce fraud, and ensure emails exist.
Phone Verification
Ensure phone numbers (domestic & international) are valid and callable. Verify country code, national prefixes, carrier data and more.
Demographic Data Enrichment
Enrich your data with B2C demographic data to gain additional insight and identify prospects like your best customers.
Firmographic Data Enrichment
Enrich your database with firmographic data, ranging from corporate to sole traders in the UK.
Geocoding
Convert addresses to latitude and longitude coordinates for better mapping and analytics.
NCOA Processing
Ensures your database remains up to date by identifying movers and updating their address information or suppressing records as needed.
Why is Data Cleansing Important?
Data cleansing, also known as data cleaning or data scrubbing, is a comprehensive process to clean, maintain and enhance your database by identifying incomplete, incorrect, inaccurate, or irrelevant parts of your data. It can be done by data quality tools that instantly verify incoming data or by batch cleansing through various data segments and reference datasets. Data cleansing is a core component of any data management strategy and should be performed often to stay on top of your customer data.

The Power of Data Cleansing
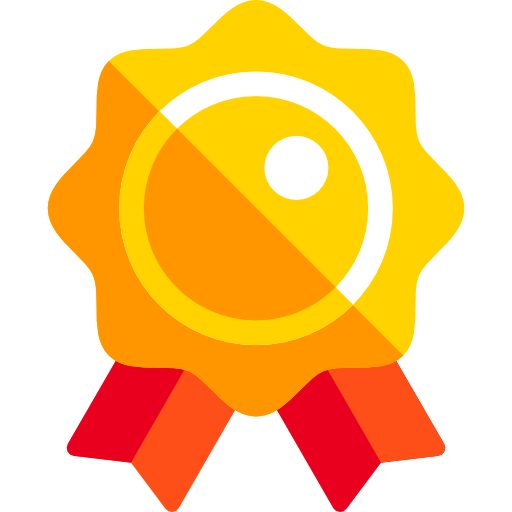
Increased Operational Efficiency
Data cleansing reduces costs, enhances automation, and optimises storage by eliminating errors and redundancies.
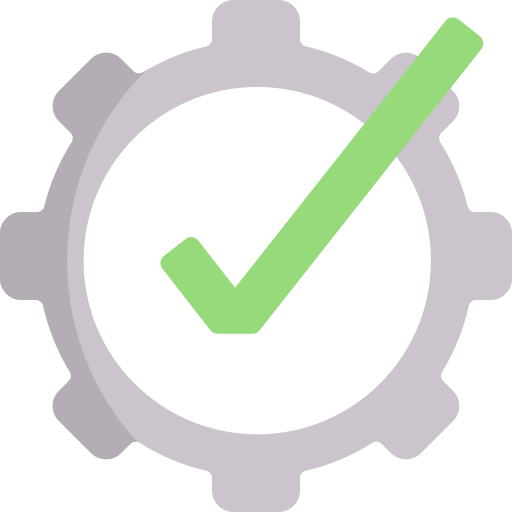
Protect Your Brand Image, Stay GDPR Compliant
Suppressing your records against gone away, MPS/TPS and deceased lists will protect your brand, keep you GDPR compliant and eliminate unnecessary costs.
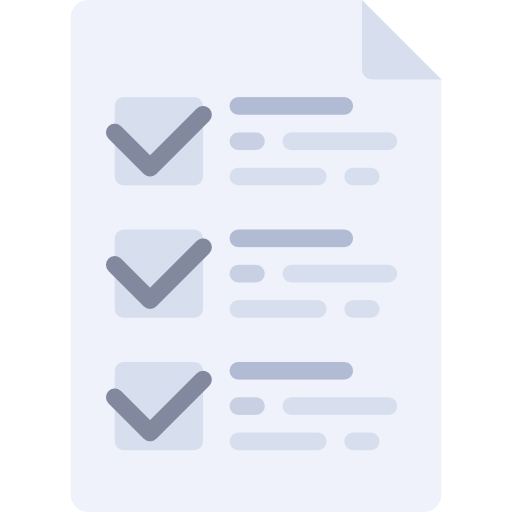
Improved Data Accuracy
Data cleansing removes duplicates, corrects errors, and ensures consistency, leading to better decisions, improved efficiency, and enhanced customer experiences.
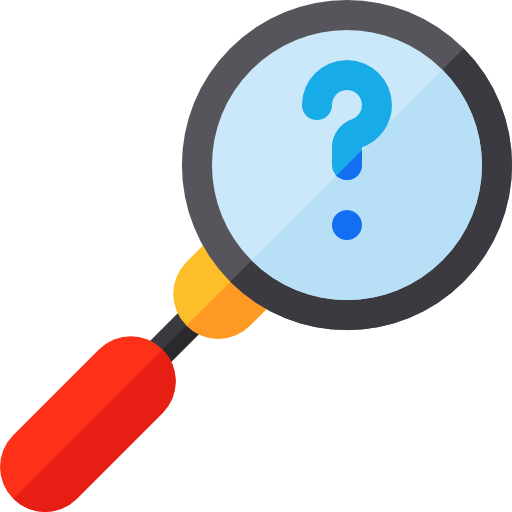
Enhanced Decision-Making
Data cleansing ensures reliable data for analytics, reduces errors, and enables accurate insights for smarter decision-making.
How to Cleanse Your Data

1 Upload
Send us your file through a secure FTP or upload via our listware portal.

2 Data Audit
Our data quality experts will audit your data to understand the current health of your prospect data.

3 Data Selection
If you know already what data quality service you want, you can select a service via listware or just let our data quality experts know.

4 Verify
Uses authoritative, in-country reference datasets to check the validity & deliverability of a physical address.

5 Transliterate
Transforms foreign languages into Latin characters so an address can easily be validated.

6 Output
A clean, deliverable address ready for mailing, order fulfilment, or analytics.
Ways to Cleanse Your Data
Common Questions About Data Cleansing
Data Cleansing is an essential step in data management and analysis, and provides the following benefits:
- Reliable and accurate analysis: Clean and accurate data is crucial for making informed business decisions and drawing reliable insights. Data cleansing ensures that the data used for analysis is free from errors and inconsistencies that could lead to incorrect conclusions or misleading results.
- Enhancing data quality: Data quality is a measure of how well data meets the requirements of its intended use. By identifying and correcting errors, such as missing values, duplicate records, or inconsistent formats, data cleansing improves data quality. High-quality data leads to better decision-making, improved operational efficiency, and increased customer satisfaction.
- Consistency and standardisation: Data cleansing helps in standardising data across different sources and systems. It involves normalising data formats, removing inconsistencies in naming conventions, and standardising units of measurement. This consistency enables data integration, comparison, and meaningful analysis across multiple datasets.
- Removal of duplicate records: Duplicates can occur in datasets due to data entry errors, system glitches, or merging of data from different sources. Data cleansing identifies and removes duplicate records, ensuring that each entity or observation is represented only once. This prevents data redundancy, reduces storage requirements, and improves data integrity.
- Compliance and regulatory requirements: Many industries are subject to regulations and compliance standards regarding data accuracy, privacy, and security. Data cleansing helps organisations comply with these requirements by ensuring that sensitive or personally identifiable information (PII) is properly handled, removing outdated or irrelevant data, and maintaining data integrity.
- Cost savings: Data cleansing can lead to cost savings by reducing unnecessary storage costs, optimising data processing and analysis, and minimising the risk of errors and inefficiencies caused by inaccurate data. Clean data streamlines business operations and supports more efficient resource allocation.
Overall, data cleansing is important because it improves the reliability, accuracy, and usefulness of data. It enables organisations to make informed decisions, gain insights, and maintain a competitive edge in today's data-driven world.
While the specific steps may vary depending on the context and the nature of the data, here are five general steps involved in data cleansing:
- Data Audit: The first step is to perform a thorough audit of the dataset to identify potential issues. This involves examining the data for missing values, duplicate records, inconsistent formats, outliers, and other anomalies. It's essential to understand the quality and structure of the data before proceeding further.
- Data Validation: In this step, the data is validated against predefined business rules or constraints. These rules define what is considered valid and meaningful data for the given context. For example, if you have a dataset of customer ages, a rule might be that the age must be a positive integer. Data that violates these rules is flagged as erroneous or suspicious.
-
Data Cleaning: Once the issues are identified, the data needs to be cleaned. This step involves various techniques such as:
- Handling Missing Data: Missing values can be imputed using methods like mean imputation, regression imputation, or deletion of incomplete records.
- Removing Duplicates: Duplicate records are identified and eliminated to avoid misleading analysis. Common approaches include comparing fields like unique identifiers or a combination of attributes to identify duplicates.
- Standardising Formats: Inconsistent formats (e.g., date formats, phone numbers, addresses) are standardised to a common format to enhance data uniformity and accuracy.
- Correcting Errors: Errors like spelling mistakes or inconsistent values are corrected based on domain knowledge or external reference sources.
- Data Transformation: Sometimes, data needs to be transformed or restructured to ensure compatibility with the desired data model or analysis requirements. This step may involve aggregating data, splitting columns, creating new features, or converting data types to facilitate subsequent data analysis or integration.
- Data Documentation and Maintenance: Finally, it is crucial to document the changes made during the data cleansing process. This documentation helps in understanding the modifications, tracking the lineage of data, and maintaining data quality over time. Additionally, regular monitoring and periodic updates are necessary to ensure the long-term integrity of the cleansed dataset.
It's important to note that data cleansing is an iterative process, and these steps may be repeated multiple times until the data is sufficiently cleaned and ready for analysis or further processing.
Data cleansing is typically performed by our data experts, who are responsible for ensuring that the data used in an organisation is accurate, consistent, and reliable. They perform various tasks to cleanse the data, such as:
- Data profiling: Analysing the data to identify inconsistencies, errors, and missing values.
- Data validation: Checking the data against predefined rules or constraints to ensure its integrity and accuracy.
- Data standardisation: Converting the data into a consistent format or structure, such as correcting spelling mistakes or abbreviations.
- Data deduplication: Identifying and removing duplicate records or entries from the dataset.
- Data normalisation: Transforming the data into a standardized format, eliminating anomalies or outliers.
- Handling missing data: Addressing missing values by either imputing them based on statistical methods or deciding on how to handle them appropriately.
- Data quality assessment: Evaluating the overall quality of the data and identifying areas for improvement.
It's important to note that data cleansing is a collaborative effort involving various stakeholders, including domain experts and IT professionals, to ensure accurate and reliable data for analysis and decision-making.
The time required for data cleansing can vary widely depending on several factors, including the size and complexity of the dataset, the quality of the initial data, the specific data cleansing tasks involved, and the tools and resources available. Here are some factors that can influence the duration of the data cleansing process:
- Dataset size: The larger the dataset, the more time-consuming the data cleansing process can be. Cleaning and processing a small dataset may take a few hours or even minutes, while cleansing a large dataset with millions of records can take days or weeks.
- Data complexity: Complex data structures or formats may require more time for cleansing. For example, unstructured or semi-structured data may require additional effort for parsing and transformation.
- Data quality: The initial quality of the data can affect the time needed for data cleansing. If the data is already relatively clean and well-structured, the cleansing process may be quicker. However, if the data is messy, inconsistent, or contains a significant number of errors, it may require more time and effort to clean.
- Data cleansing tasks: The specific tasks involved in data cleansing can vary. Some tasks, such as removing duplicates or standardising formats, may be relatively quick, while others, like imputing missing values or performing complex data transformations, can be more time-consuming.
- Tools and resources: The choice of data cleansing tools and the availability of resources can impact the timeline. More sophisticated tools with automation capabilities can speed up the process. Additionally, the availability of skilled data analysts or data engineers can influence the speed of data cleansing.
It's challenging to provide an exact time frame for data cleansing as it varies significantly depending on the factors mentioned above. It is recommended to perform a thorough analysis of the dataset and consider the complexity of the required cleansing tasks to accurately estimate the time needed.
Data cleansing, or data cleaning, offers several benefits for organisations that rely on data for decision-making, analysis, and operations. Here are some key benefits of data cleansing:
- Improved Data Accuracy: Data cleansing helps identify and rectify inaccuracies, errors, and inconsistencies in the dataset. By removing duplicate entries, correcting misspellings, and standardising formats, data cleansing enhances data accuracy. Clean and accurate data ensures that the organisation's analyses and decisions are based on reliable information.
- Enhanced Data Quality: Data cleansing improves the overall quality of the dataset. By eliminating errors, inconsistencies, and outliers, it ensures that the data is consistent, complete, and reliable. Clean data enhances the effectiveness of data analytics, reporting, and data-driven processes.
- Increased Operational Efficiency: Clean data leads to improved operational efficiency. It reduces the time spent on data troubleshooting, error handling, and data-related issues. With accurate and reliable data, organisations can make better-informed decisions and execute processes more efficiently.
- Better Decision-Making: Data cleansing contributes to better decision-making. Clean data provides a more accurate and comprehensive view of the business operations, customer behaviour, market trends, and other critical factors. This enables organisations to make data-driven decisions with greater confidence, leading to improved outcomes.
- Enhanced Customer Insights: Clean data allows for a more accurate analysis of customer information, leading to better insights. It enables organisations to understand customer preferences, behaviour patterns, and segmentation more effectively. Clean data supports targeted marketing campaigns, personalised customer experiences, and improved customer satisfaction.
- Regulatory Compliance: Data cleansing helps organisations maintain compliance with data protection regulations, privacy laws, and industry standards. By ensuring the accuracy and integrity of data, organisations can mitigate the risks associated with non-compliance, avoid penalties, and protect sensitive information.
- Cost Savings: Data cleansing can lead to cost savings in various ways. By identifying and removing duplicate records, organisations can reduce storage costs. It also minimises the time and effort spent on manual data troubleshooting and error correction. Moreover, clean data supports more efficient business processes, optimising resource allocation and reducing operational costs.
Overall, data cleansing plays a crucial role in improving data quality, accuracy, and reliability, leading to more informed decision-making, improved operational efficiency, and enhanced business performance.
The frequency of data cleansing depends on several factors, including the nature of the data, the rate of data change, the importance of data accuracy, and the specific requirements of the organisation. While there is no one-size-fits-all answer, here are some considerations for determining how often data cleansing should be performed:
- Data Change Rate: If the data in your organisation's systems undergoes frequent updates, additions, or deletions, it may require more frequent data cleansing. Regularly changing data can introduce errors, duplicates, or inconsistencies that need to be addressed promptly. Monitoring data quality and performing cleansing routines more frequently can help maintain data accuracy.
- Business Needs and Criticality: Consider the impact of data accuracy on your organisation's operations, decision-making, and customer experience. If data integrity is critical for your business, such as those in the financial or healthcare industries, more frequent data cleansing is necessary to ensure reliable insights and compliance with regulations.
- Data Source and Integration Points: If your organisation collects data from multiple sources or integrates data from external systems, there may be a need for more frequent data cleansing. Different sources may have varying data quality, and regular cleansing can help maintain consistency and accuracy throughout the integrated dataset.
- Seasonality and Events: Some industries or businesses experience seasonal fluctuations or events that impact data quality. For example, retail businesses may require more frequent data cleansing during peak shopping seasons, while tax-related data may need to be cleansed before specific deadlines. Consider such events or patterns that may require additional data cleansing efforts.
- Data Governance Policies: Organisations that have established data governance policies and frameworks may define the frequency of data cleansing as part of their data management practices. These policies can help guide the regular assessment and cleansing of data to maintain quality standards.
It is recommended to establish a data cleansing schedule based on a combination of the factors mentioned above. Regular monitoring of data quality and conducting periodic data audits can help identify patterns, trends, or issues that inform the frequency of data cleansing. Adjust the frequency as needed to ensure that the data remains accurate, reliable, and aligned with your business requirements.
When undertaking data cleansing, businesses should consider several factors to ensure a successful and effective process. Here are some key considerations:
- Define Objectives: Clearly define the objectives of the data cleansing initiative. Identify the specific problems or issues you want to address, such as removing duplicates, standardising formats, or resolving inconsistencies. Having clear objectives helps focus the cleansing efforts and measure the success of the process.
- Data Quality Assessment: Assess the current state of data quality in your organisation. Understand the strengths and weaknesses of your data, identify areas of improvement, and prioritise the data cleansing tasks accordingly. Data profiling and analysis can provide valuable insights into data quality issues.
- Data Governance: Consider the data governance policies and procedures in place within your organisation. Data cleansing should align with the overall data governance framework, ensuring that data quality standards, ownership, and responsibilities are clearly defined and followed.
- Data Cleansing Strategy: Develop a data cleansing strategy that outlines the approach, methodologies, and tools to be used. Determine the sequence of cleansing tasks, establish rules and criteria for data validation, standardisation, and deduplication. Consider the resources, budget, and timelines required for the cleansing process.
- Data Backup and Security: Before performing data cleansing, ensure that appropriate data backup mechanisms are in place. This safeguards against any unintended data loss or corruption during the cleansing process. Also, maintain data security protocols to protect sensitive information during and after the cleansing activities.
- Stakeholder Collaboration: Involve relevant stakeholders in the data cleansing process. Collaborate with data owners, domain experts, IT teams, and business users to gain their insights, validate data, and ensure the accuracy and relevance of the cleansing activities. Their knowledge and input can significantly enhance the effectiveness of the process.
- Data Cleansing Tools and Resources: Evaluate and select appropriate data cleansing tools that align with your requirements. Consider factors such as scalability, ease of use, compatibility with data sources, and automation capabilities. Ensure that you have the necessary resources, including skilled data analysts or engineers, to execute the cleansing tasks effectively.
- Documentation and Auditing: Document the data cleansing process, including the steps taken, transformations applied, and decisions made. Maintain an audit trail to track changes and ensure transparency. Documentation helps in future reference, compliance, and continuous improvement of data quality practices.
- Monitoring and Maintenance: Data cleansing is not a one-time activity. Establish mechanisms for ongoing monitoring of data quality and plan for regular maintenance. Continuously assess and address new data quality issues that may arise due to changes in data sources, business requirements, or technology.
By considering these factors, businesses can approach data cleansing in a structured and comprehensive manner, leading to improved data quality, accuracy, and reliability.
Data cleansing is suitable for a wide range of organisations and industries that work with data. Here are some examples of who can benefit from data cleansing:
- Businesses of all Sizes: Whether it's small startups or large enterprises, businesses of all sizes can benefit from data cleansing. Regardless of the volume of data they handle, maintaining accurate and reliable data is crucial for informed decision-making, operational efficiency, and customer satisfaction.
- Data-Driven Companies: Organisations that heavily rely on data for their operations, such as e-commerce companies, financial institutions, marketing agencies, and healthcare providers, can greatly benefit from data cleansing. Clean and high-quality data ensures accurate analyses, personalised customer experiences, risk mitigation, and compliance with industry regulations.
- Industries with Complex Data: Industries dealing with complex data, such as manufacturing, supply chain management, logistics, and utilities, often face challenges of data inconsistency, errors, and duplicates. Data cleansing helps improve the reliability of data, optimise processes, and ensure the smooth functioning of operations.
- Customer-Centric Businesses: Organisations that prioritise delivering exceptional customer experiences can benefit from data cleansing. By maintaining accurate customer data, businesses can gain insights into customer behaviour, preferences, and trends, enabling targeted marketing, personalised interactions, and improved customer satisfaction.
- Data Warehousing and Analytics: Data cleansing is crucial for organisations that build data warehouses or utilize analytics platforms. Clean and consistent data is essential for accurate reporting, data visualisation, predictive modelling, and other data-driven initiatives.
- Compliance-Driven Industries: Industries with strict regulatory requirements, such as healthcare, finance, and legal sectors, need to ensure data accuracy and integrity. Data cleansing helps maintain compliance with regulations, privacy laws, and security standards, reducing the risk of penalties and reputational damage.
- Mergers and Acquisitions: Organisations involved in mergers, acquisitions, or data integration projects can benefit from data cleansing. It helps harmonise and consolidate disparate data sources, resolve data inconsistencies, and establish a unified and accurate dataset across the merged or integrated entities.
In summary, data cleansing is suitable for any organisation that values data accuracy, reliability, and the ability to make informed decisions based on high-quality data. It is particularly beneficial for businesses that heavily rely on data, deal with complex data structures, operate in compliance-driven industries, or aim to enhance customer experiences.
More on Data Cleansing
Explore our success stories, articles and expert insights on the power and benefits of data cleansing.
Ready to Start Your Demo?
Start today with Melissa's wide range of Data Quality Solutions, Tools, and Support.
Schedule a Demo